The numerator of the F-test, for the hypothesis you want to test, should be based on the adjusted SS's that is last in the sequence or is obtained from the adjusted sums of squares. That will be very close to what you would get using the approximate method we mentioned earlier. The general linear test is the most powerful test for this type of situation with unbalanced data.
Model
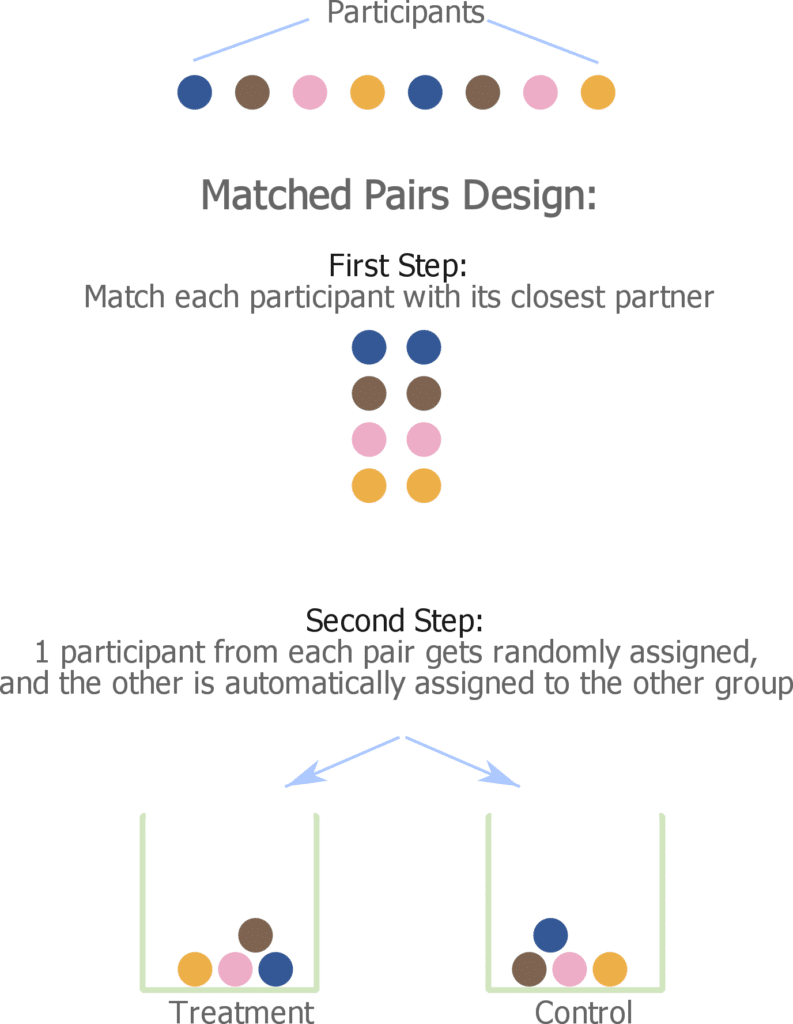
The row effect is the order of treatment, whether A is done first or second or whether B is done first or second. So, if we have 10 subjects we could label all 10 of the subjects as we have above, or we could label the subjects 1 and 2 nested in a square. This is similar to the situation where we have replicated Latin squares - in this case five reps of 2 × 2 Latin squares, just as was shown previously in Case 2. We want to account for all three of the blocking factor sources of variation, and remove each of these sources of error from the experiment.
Mean Squares
Sign up for the DDIY Newsletter and never miss an update on the best business tools and marketing tips. We provide advice and reviews to help you choose the best people and tools to grow your business. Here are some of the recent trends that have taken over the graphic design scene and helped many businesses see success in their marketing campaigns. With so many people consuming thousands of advertisements a day, your marketing strategy must include eye-catching designs that will pull consumers in. Graphic design and marketing are heavily linked to each other, with the former often determining the latter’s success.
ANOVA Display for the RCBD
We can test for row and column effects, but our focus of interest in a Latin square design is on the treatments. Just as in RCBD, the row and column factors are included to reduce the error variation but are not typically of interest. And, depending on how we've conducted the experiment they often haven't been randomized in a way that allows us to make any reliable inference from those tests.
Blocks are usually treated as random effects, as they would represent the population of all possible blocks. In other words, the mean comparison among blocks is not of interest. But the variation between blocks has to be incorporated into the model and will be partitioned out of the Error Mean squares of the CRD, resulting in a smaller MSE for testing hypotheses about treatments. Here, the number of rows to be specified is our block size (and number of treatment levels), which yields a random assignment from Block 1.
A Guide on Data Analysis
Inside TurboTax's 20-Year Fight to Stop Americans From Filing Their Taxes for Free - ProPublica
Inside TurboTax's 20-Year Fight to Stop Americans From Filing Their Taxes for Free.
Posted: Thu, 17 Oct 2019 07:00:00 GMT [source]
Driving experience in this case can be used as a blocking variable. We will then divide up the participants into multiple groups or blocks, so that those in each block share similar driving experiences. For example, let's say we decide to place them into three blocks based on driving experience - seasoned; intermediate; inexperienced.
Table
If we add subjects in sets of complete Latin squares then we retain the orthogonality that we have with a single square. The degrees of freedom for error grows very rapidly when you replicate Latin squares. But usually if you are using a Latin Square then you are probably not worried too much about this error. The error is more dependent on the specific conditions that exist for performing the experiment. It depends on the conditions under which the experiment is going to be conducted.
Therefore, one can test the block simply to confirm that the block factor is effective and explains variation that would otherwise be part of your experimental error. However, you generally cannot make any stronger conclusions from the test on a block factor, because you may not have randomly selected the blocks from any population, nor randomly assigned the levels. Formal test of interaction effects between blocks and treatments for a randomized block design. Can also considered for testing additivity in 2-way analyses when there is only one observation per cell. What we now realize is that two blocking factors is not enough!
Block a few of the most important nuisance factors
This gives us a design where we have each of the treatments and in each row and in each column. The original use of the term block for removing a source of variation comes from agriculture. If the section of land contains a large number of plots, they will tend to be very variable - heterogeneous. An alternate way of summarizing the design trials would be to use a 4x3 matrix whose 4 rows are the levels of the treatment X1 and whose columns are the 3 levels of the blocking variable X2.
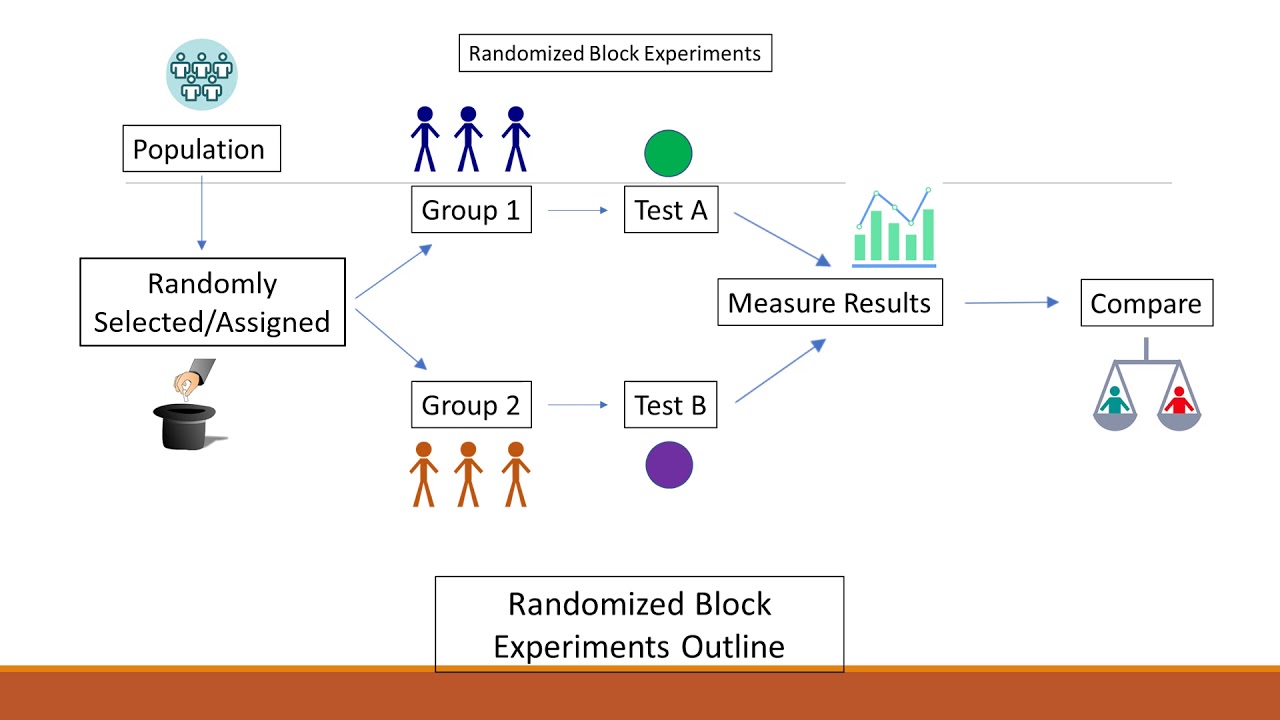
An assumption that we make when using a Latin square design is that the three factors (treatments, and two nuisance factors) do not interact. If this assumption is violated, the Latin Square design error term will be inflated. The RCBD utilizes an additive model – one in which there is no interaction between treatments and blocks. The error term in a randomized complete block model reflects how the treatment effect varies from one block to another. The partitioning of the variation of the sum of squares and the corresponding partitioning of the degrees of freedom provides the basis for our orthogonal analysis of variance. By extension, note that the trials for any K-factor randomized block design are simply the cell indices of a k dimensional matrix.
The cells in the matrix have indices that match the X1, X2 combinations above. Gender is a common nuisance variable to use as a blocking factor in experiments since males and females tend to respond differently to a wide variety of treatments. If the number of times treatments occur together within a block is equal across the design for all pairs of treatments then we call this a balanced incomplete block design (BIBD). Here is an actual data example for a design balanced for carryover effects.
If we conduct this as a blocked experiment, we would assign all four tips to the same test specimen, randomly assigned to be tested on a different location on the specimen. Since each treatment occurs once in each block, the number of test specimens is the number of replicates. Many times there are nuisance factors that are unknown and uncontrollable (sometimes called a “lurking” variable).
A nuisance factor is a factor that has some effect on the response, but is of no interest to the experimenter; however, the variability it transmits to the response needs to be minimized or explained. We will talk about treatment factors, which we are interested in, and blocking factors, which we are not interested in. We will try to account for these nuisance factors in our model and analysis.
No comments:
Post a Comment